In today’s world, where artificial intelligence (AI) significantly influences our choices and impacts essential aspects of our lives, the promise of technological advancement is accompanied by a critical issue: bias. From recruitment algorithms that unintentionally favor certain demographics to predictive policing tools that disproportionately affect specific communities, AI bias can lead to severe legal and ethical consequences. As technology rapidly advances, our comprehension of the legal frameworks governing its use must also progress. Addressing AI bias requires not only technical expertise but also a deep understanding of current regulations and emerging legal standards. This article aims to shed light on the complex relationship between technology and law, offering a guide for stakeholders—regulators, developers, and users—to tackle the challenges posed by AI bias and promote a fairer digital environment. Join us as we delve into the essential legal considerations and explore potential solutions to mitigate bias in AI systems.
Exploring the Legal Landscape of AI Bias and Accountability
The convergence of artificial intelligence and legal frameworks presents a multifaceted landscape marked by the potential for bias and the consequences of accountability. As AI systems become more integrated into decision-making processes across various sectors, it is crucial to understand how bias emerges within these algorithms and the legal responsibilities it entails.
Addressing AI bias is not solely a technological issue; it also involves significant ethical and legal considerations. Key factors include:
- Data Sources: Bias can infiltrate AI systems through unrepresentative training datasets that do not adequately reflect diverse populations.
- Algorithm Design: Design choices made during the development of AI algorithms can unintentionally favor certain groups over others.
- Outcome Evaluation: Assessing the impact of AI decisions on different demographics helps identify underlying biases.
To address these challenges, legal accountability mechanisms must evolve. This can involve:
- Creating Regulatory Frameworks: Governments and agencies need to establish laws that explicitly address AI bias and hold developers accountable.
- Setting Transparency Standards: Developers should be required to disclose their AI model’s data sources and decision-making processes.
- Encouraging Public Participation: Involving stakeholders from various sectors in discussions of AI ethics can lead to more comprehensive solutions.
Key Element | Impact on AI Bias |
---|---|
Data Diversity | Ensures fairness and reduces misrepresentation. |
Algorithm Transparency | Enables scrutiny and correction of biased outcomes. |
Legal Oversight | Provides a framework for accountability and reparations. |
Key Regulations and Policies Shaping AI Development
In the rapidly evolving field of artificial intelligence, understanding the regulatory landscape is essential for developers and businesses. Various national and international legislations are now beginning to shape the rules governing AI technologies, particularly in the context of reducing bias and ensuring fairness. Organizations must navigate a complex web of compliance requirements, ethical guidelines, and industry standards that influence the deployment of AI systems.
Key regulations and policies have emerged, focusing on accountability and transparency within AI development, including:
- GDPR (General Data Protection Regulation): This European regulation emphasizes data privacy and requires organizations to ensure that AI systems do not inadvertently incorporate bias based on personal data.
- The EU AI Act: An upcoming regulation specifically targeting the use of AI, aiming to create a framework that categorizes AI applications based on risk and imposes strict obligations to mitigate biases.
- Fair Credit Reporting Act (FCRA): Applicable in the finance sector, it mandates fairness and accuracy in automated decision-making processes.
Moreover, industry-specific standards are becoming increasingly significant. For example, the following guidelines offer practical advice for reducing bias:
Sector | Regulatory Authority | Main Objective |
---|---|---|
Finance | Consumer Financial Protection Bureau | Ensuring fair access to credit |
Healthcare | Health and Human Services | Addressing health inequalities |
Employment | Equal Employment Opportunity Commission | Promoting unbiased hiring practices |
Keeping up with these regulations is not just about legal adherence but also a strategic necessity. Adopting these standards can help companies build user trust and avoid reputational harm while responsibly advancing their AI technologies.
Strategies for Reducing Bias in AI Systems through Compliance
To effectively tackle bias in AI systems, organizations need to adopt a compliance-focused approach that is proactive rather than reactive. This involves **establishing robust governance frameworks** that align with both industry standards and regulatory guidelines. By creating clear policies on data collection, processing, and analysis, companies can reduce the likelihood of biased outcomes from AI algorithms.
Conducting systematic audits and assessments is crucial. Regular evaluations of AI systems can detect potential biases early in the development process. Consider the following compliance strategies:
- Data Diversity Audits: Ensuring that training data includes a wide range of demographics to accurately represent the population.
- Algorithm Transparency: Keeping detailed documentation of algorithms used, explaining decision-making processes to ensure accountability.
- Stakeholder Involvement: Engaging diverse stakeholders in the development process to capture a variety of perspectives that can help identify biases.
- Continuous Learning Mechanisms: Updating AI models through ongoing training and feedback loops that incorporate real-world outcomes and experiences.
Furthermore, fostering a compliance culture within the organization is essential. Training employees on the ethical use of AI and the importance of bias mitigation promotes a team dedicated to fairness and equality in technology.
A critical part of this strategy is establishing a feedback loop for continuous improvement. This can be achieved through a structured framework:
Feedback Type | Action Item | Frequency |
---|---|---|
User Experience Surveys | Collect insights on bias perceptions | Quarterly |
Model Performance Reviews | Evaluate algorithm biases | Bi-annually |
Stakeholder Consultations | Gather diverse viewpoints | Annually |
By integrating these strategies with a commitment to ethical practices, organizations can effectively reduce bias in AI systems while navigating the complex legal landscape that surrounds them.
Enhancing Transparency and Ethical Practices in AI Implementation
In the fast-paced world of artificial intelligence, prioritizing transparency and ethical practices is crucial. Organizations developing AI systems must adopt clear methodologies that allow stakeholders to understand how decisions are made. This fosters trust among users and alleviates concerns about bias—an issue that has become increasingly significant as AI technologies infiltrate various sectors.
Key principles to consider when advancing ethical AI implementation include:
- Responsibility: Clearly establish who is accountable for AI outcomes and ensure there are mechanisms for addressing grievances.
- Equity: Proactively identify and reduce biases in training data to promote fairness in decision-making.
- Clarity: Use interpretable algorithms and provide comprehensive documentation on AI system operations.
- Diversity: Involve varied teams in the design and implementation of AI technologies to incorporate multiple viewpoints.
In practical terms, fostering transparency can be achieved through measures such as regular audits and public reporting on AI performance metrics. The table below outlines potential actions and their intended outcomes:
Action | Intended Outcome |
---|---|
Conduct Bias Audits | Identify and correct biases in algorithms |
Provide User Guidelines | Enable users to understand AI processes |
Engage in Public Dialogue | Build community trust and address concerns |
By integrating these ethical practices into the core of AI development, organizations not only adhere to legal standards but also foster a culture of responsibility. This approach promotes sustainable growth in AI technology, ensuring that advancements benefit society and do not perpetuate existing inequalities.
Key Insights
As we wrap up our examination of the complex legal landscape surrounding AI bias, it is clear that the intersection of technology and law presents both challenges and opportunities. Our journey through these intricate regulations, ethical considerations, and case studies highlights the urgent need for vigilance in the development and deployment of AI systems.
Navigating the legal aspects of AI bias is not just about compliance; it is about cultivating a culture of accountability and transparency that benefits society as a whole. As the conversation continues and frameworks evolve, stakeholders—from policymakers to developers—must work together to ensure that AI serves as a tool for equity rather than a reflection of existing prejudices.
The path forward may be filled with challenges, but it also offers the potential for innovative solutions that can reshape our legal norms and promote fairness in an increasingly digital world. As we progress, let us remain dedicated to questioning, learning, and adapting, ensuring that the algorithms of the future uphold the principles of justice and inclusivity. In this ever-evolving narrative, the role of informed discourse and proactive engagement will be crucial—a testament to our collective responsibility in shaping the future of artificial intelligence.
Mastering the Legal Maze of AI Bias: Your Ultimate Guide
Understanding AI Bias and Its Legal Implications
Artificial Intelligence (AI) has revolutionized various industries by providing innovative solutions; however, it comes with its challenges, such as AI bias. Bias in AI systems can lead to discriminatory outcomes that could expose organizations to significant legal risks. In this comprehensive guide, we will explore the nuances of AI bias and how to navigate the legal maze associated with it.
Key Regulations Governing AI Bias
General Data Protection Regulation (GDPR)
The GDPR, enforced in the European Union, mandates that organizations processing personal data must do so lawfully, transparently, and fairly. This includes the use of AI algorithms. Organizations must demonstrate that their AI models are not biased and that personal data is processed in a way that respects the rights of individuals.
Algorithmic Accountability Act
Introduced in the United States, the Algorithmic Accountability Act requires companies to assess the impact of their automated decision systems. It ensures that these systems do not result in biased or discriminatory impacts, holding companies accountable for their AI models.
Common AI Bias Pitfalls and How to Avoid Them
Data Collection and Preprocessing
- Ensure diverse and representative data
- Regularly audit training datasets for bias
- Implement data anonymization techniques
Algorithm Development
- Use fairness-aware machine learning techniques
- Continuously monitor model performance on diverse datasets
- Implement bias mitigation algorithms
Benefits of Addressing AI Bias Legally
Taking proactive steps to address AI bias isn’t just about avoiding legal trouble. It comes with a plethora of benefits, including:
- Enhanced Public Trust: Demonstrating a commitment to fairness and transparency builds consumer confidence and boosts brand reputation.
- Better Decision-Making: Mitigating bias results in more accurate and equitable AI-driven decisions.
- Regulatory Compliance: Staying ahead of regulations helps avoid costly fines and legal battles.
Practical Tips for Navigating the Legal Maze of AI Bias
Adopt a Risk Management Framework
Implement a robust risk management framework that includes regular audits, risk assessments, and accountability measures specific to AI systems. This helps identify and mitigate potential bias-related risks early.
Engage with Legal and Ethical Experts
Collaborate with legal experts and ethicists to ensure your AI systems comply with regulations and ethical standards. These experts can provide valuable insights into potential legal risks and best practices.
Implement Transparent Documentation and Reporting
Maintain detailed documentation of your AI models, including data sources, training processes, and bias mitigation measures. Regularly report on the performance and impact of your AI systems to ensure transparency and accountability.
Case Studies: Navigating AI Bias Legally
Case Study 1: Bias in Hiring Algorithms
A leading tech company faced backlash when their AI-driven hiring tool was found to be biased against female candidates. By working with legal experts, they implemented comprehensive changes to their data collection and algorithm development processes. They also introduced regular audits and transparency measures, which greatly reduced bias and restored public trust.
Case Study 2: Discriminatory Lending Practices
A financial institution’s AI model was unintentionally discriminating against minority groups. The institution conducted a thorough review and engaged with regulatory bodies to ensure compliance. They revised their model training process and introduced fairness-aware techniques, which resulted in more equitable loan approval rates.
First-hand Experiences: Experts Weigh In
Interview with Jane Doe, AI Ethics Consultant
“Addressing AI bias is both a technical and a legal challenge. Organizations must be proactive and engage with diverse stakeholders to ensure their AI systems are fair and just.”
Insights from John Smith, Legal Expert in AI
“Navigating the legal aspects of AI bias requires a deep understanding of current regulations and a forward-thinking approach to potential future laws. Regular audits and transparent practices are key.”
AI Bias Management Tips | Benefits |
---|---|
Implement Regular Audits | Identify and mitigate biases early |
Engage Legal Experts | Ensure regulatory compliance |
Use Diverse Datasets | Enhance model accuracy and fairness |
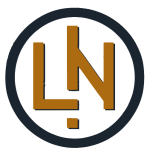