In the swiftly advancing realm of technology, artificial intelligence (AI) is leading the charge, poised to transform industries, expand human capabilities, and revolutionize our interactions with the world. However, as we venture further into the era of intelligent machines, the legal ramifications of AI development are becoming increasingly intricate and pressing. From intellectual property and accountability issues to ethical dilemmas and regulatory hurdles, the intersection of law and AI raises numerous questions that require thorough examination. As innovators seek to leverage AI’s potential, navigating this complex legal landscape is crucial not only for compliance but also for fostering a responsible and equitable technological future. This article delves into the primary legal challenges in AI development, illuminating the intricate web of laws, regulations, and ethical considerations that will shape the future of this groundbreaking field.
Intellectual Property Rights in AI Innovations
As technology progresses, so does the intricate landscape of intellectual property (IP) rights associated with artificial intelligence. Developers and innovators face unique challenges in safeguarding their creations, which often integrate algorithms, data, and user interactions. Understanding how existing IP laws, such as copyright, patents, and trade secrets, apply to AI technologies is essential.
Key considerations for navigating IP rights in the AI domain include:
- Copyright Issues: While software code is generally protected under copyright law, the question arises: Can AI-generated content be copyrighted? The lack of a human creator complicates matters, leading to debates about ownership and residual rights.
- Patent Protection: Innovations in AI can be patentable, but they must meet the criteria of novelty, non-obviousness, and utility. Documenting the development process meticulously is crucial to establish precedence and defend against potential claims.
- Trade Secrets: Many companies rely on trade secret protection for their algorithms and models. However, this requires robust security measures to prevent reverse engineering or unintended disclosure.
Additionally, a growing debate centers around the legal status of AI systems themselves. The question of whether AI can be an inventor under patent laws, for example, has seen varying interpretations across jurisdictions. Below is a comparative table that highlights the current landscape of IP protection in various regions:
Region | Copyright for AI-Generated Work | Patent Recognition for AI | Trade Secret Emphasis |
---|---|---|---|
United States | Limited; human authors required | Possible, but criteria strict | Strong protections available |
European Union | Potentially; ongoing debates | Allows AI as inventor in some cases | Robust framework in place |
China | Emerging perspectives | Active in recognizing AI inventions | Prioritized for tech firms |
Effectively protecting AI innovations requires a proactive approach. Engaging legal experts early in the development process, continuously monitoring changes in IP law, and considering collaborative approaches to IP management will be indispensable for ensuring your AI creations are safeguarded.
Ethical Concerns and Accountability in AI Systems
As the integration of artificial intelligence into various sectors deepens, the ethical implications surrounding its development and use have become increasingly pronounced. Given the potential for AI systems to impact lives on multiple levels, the industry must prioritize a framework that ensures ethical practices and accountability. Key considerations include:
- Transparency: AI algorithms often operate as “black boxes,” making their decision-making processes obscure. Developers should strive for greater transparency, allowing users and stakeholders to understand how and why decisions are made.
- Bias Mitigation: AI systems can perpetuate or even exacerbate existing biases if trained on skewed datasets. Ensuring a diverse range of data and incorporating bias detection tools during development can help minimize discrimination.
- User Consent: Collecting data for AI training should be conducted under clear terms and explicit consent from users, empowering them with control over their personal information.
Moreover, establishing accountability measures is pivotal in the AI landscape. Developers and organizations must define who is liable in instances where AI systems produce harmful outcomes or violate ethical standards. Implementing structured oversight and regulatory compliance can bolster responsible AI deployment. To clarify these roles, the following table outlines potential parties involved in AI accountability:
Role | Responsibility |
---|---|
Developers | Ensuring ethical design and implementation |
Organizations | Maintaining oversight and compliance |
Regulators | Enforcing laws and guidelines |
as AI continues to evolve and integrate into various aspects of society, addressing the legal and ethical challenges it presents is crucial. By proactively engaging with these issues, we can ensure that AI development not only complies with existing laws but also promotes a fair and responsible technological future.
By addressing these crucial aspects, stakeholders in the AI ecosystem can cultivate an environment that not only mitigates ethical concerns but also fosters trust and accountability. The future of AI development hinges on the commitment to responsible practices, ensuring that advancements benefit society in an equitable manner.
Ensuring Compliance: Regulatory Frameworks for AI Development
As the rapid advancement of artificial intelligence technology continues to reshape industries and societies, the need for a robust regulatory framework has never been more urgent. Ensuring compliance with existing laws while fostering innovation requires a delicate balance. Key stakeholders, including governments, industry leaders, and civil society, must collaborate to develop guidelines that address the complexities of AI development. This collaborative effort is essential to mitigate risks and safeguard public interests.
To navigate the evolving landscape of AI regulation, certain foundational principles must be established. These could include:
- Transparency: AI systems should be designed to operate transparently, allowing users to understand how decisions are made.
- Accountability: Developers and organizations must be held accountable for the ethical implications and impacts of their AI systems.
- Fairness: Regulatory frameworks should seek to prevent discrimination and bias in AI algorithms, promoting equitable outcomes for all users.
- Privacy: Striking a balance between leveraging data for AI advancements and protecting individual privacy rights is crucial.
Multiple jurisdictions are already taking steps towards formalizing regulations tailored for artificial intelligence. For example, the European Union’s proposed AI Act presents a framework that categorizes AI systems by risk level, enabling targeted regulatory responses. Below is a simple overview of the risk categories:
Risk Level | Description | Examples |
---|---|---|
Minimal Risk | AI applications with low potential for harm | Chatbots for customer service |
Limited Risk | AI with some risks requiring transparency | Recommendation systems |
High Risk | Significant impact on rights and safety | Facial recognition, autonomous vehicles |
Unacceptable Risk | AI posing a threat to safety, freedoms, or rights | Social scoring systems |
As nations formulate their policies, the interplay between local regulations and global standards will dictate the future landscape of AI. The emergence of international agreements and collaborative frameworks could pave the way for a universally accepted model, ensuring that AI development aligns with shared ethical standards. This interplay will not only influence compliance but also shape the very nature of innovation in artificial intelligence.
Mitigating Bias and Promoting Transparency in Machine Learning Models
As the field of artificial intelligence continues to evolve, the significance of fairness and accountability in machine learning models cannot be overstated. Bias can inadvertently seep into algorithms, often reflecting historical injustices or societal stereotypes, thus amplifying inequality in various domains. To combat this, developers and researchers are adopting multiple strategies aimed at ensuring that machine learning systems operate in an equitable manner.
- Data Audits: Conducting comprehensive reviews of training datasets to identify and rectify any imbalances or harmful stereotypes is essential. This ensures that models learn from a diverse array of inputs, minimizing the potential for discriminatory outcomes.
- Algorithmic Transparency: Developing frameworks that allow stakeholders to understand how models reach their conclusions. By providing clear documentation and rationales for decisions made by algorithms, users can gain confidence in their reliability and Ongoing Model Assessments: Regularly evaluating and retraining models is essential to ensure they adapt to evolving social contexts. Setting performance and fairness benchmarks can aid in tracking their continuous effectiveness and equity.
Additionally, fostering a culture of collaboration and inclusivity within the AI community is crucial. Engaging diverse teams can help identify blind spots in model development and drive innovation. Enhanced collaboration across various fields—such as law, ethics, and social sciences—can offer valuable insights into the real-world implications of deploying AI technologies.
Strategy | Description |
---|---|
Data Audits | Identify and correct biases in training datasets. |
Algorithmic Transparency | Clarify and make the decision-making process of algorithms understandable. |
Regular Evaluations | Continuously assess models for fairness and performance. |
Diverse Collaboration | Engage interdisciplinary teams to address AI’s societal impact. |
Implementing these practices is not merely a regulatory requirement but also a moral duty. By prioritizing fairness and transparency, artificial intelligence can become a tool that upholds human rights and trust in technology, avoiding the pitfalls of bias and discrimination.
Concluding Thoughts
As we stand at the intersection of innovation and regulation, the development of artificial intelligence calls for a reevaluation of the frameworks that govern technology and ethics. The legal issues surrounding AI are intricate, multifaceted, and constantly evolving, much like the technology itself. As we delve deeper into an era where machines learn and adapt, it is crucial to address these challenges with a balanced approach—one that fosters creativity and progress while safeguarding human rights and societal values.
The journey toward a responsible AI landscape will require collaboration among technologists, policymakers, and ethicists, forging a path that respects the nuances of both law and human experience. By adopting a proactive stance, we can harness the potential of artificial intelligence to enrich our lives while ensuring that accountability and transparency remain at the forefront of this exciting evolution.
As we navigate the legal complexities of AI development, let us remember: the goal is not just to create intelligent systems, but to develop a framework where those systems can thrive in harmony with the society they serve. Together, we can shape a future where technology enhances the human experience, ethically and responsibly. The challenge is significant, but so too is the opportunity—let’s rise to meet it.
Navigating the Legal Maze of Artificial Intelligence Development
Artificial Intelligence (AI) development is rapidly transforming industries, but navigating the legal landscape can be daunting. Discover the key regulations, compliance strategies, and real-world case studies essential for successful AI development.
Understanding AI Regulations
Global Legislation and Compliance
The regulatory environment for AI varies across different regions. Understanding these regulations is crucial for compliance and to avoid potential legal pitfalls.
United States
In the US, AI is subject to various federal and state laws, including data privacy regulations like CCPA (California Consumer Privacy Act) and laws governing specific industries, such as health and finance.
European Union
The EU’s approach to AI regulation focuses on ethics and human rights. The General Data Protection Regulation (GDPR) has stringent requirements for data protection, impacting AI development significantly.
China
China’s AI regulations are driven by its national strategy to dominate AI technology. The country has issued guidelines emphasizing security, accountability, and privacy in AI systems.
Region | Key Regulation | Main Focus |
---|---|---|
United States | CCPA | Data Privacy |
European Union | GDPR | Data Protection |
China | National AI guidelines | Security and Privacy |
Legal Challenges in AI Development
Intellectual Property and AI
Intellectual Property (IP) laws can be tricky when it comes to AI, from ownership of AI-generated works to patenting AI inventions. Understanding IP rights is crucial to protecting your AI innovations.
Ownership of AI-Generated Content
Who owns the content created by AI? This question is increasingly relevant as AI systems produce creative outputs. Most jurisdictions currently attribute ownership to the human creator or the entity directing the AI.
Patentability of AI Inventions
Getting patents for AI can be challenging due to issues of originality and non-obviousness. Countries like the US and the EU are still refining their approaches to AI patents.
Data Privacy and Security
AI systems often rely on vast amounts of data, raising significant data privacy and security concerns. Ensuring compliance with regulations like GDPR and CCPA is essential to avoid fines and legal problems.
Liability and Accountability
Determining liability for actions taken by AI systems is complex. Different scenarios, such as autonomous vehicles or AI in healthcare, each have unique considerations for liability and accountability.
Autonomous Vehicles
In the case of autonomous vehicles, who is liable for accidents—the manufacturer, the software developer, or the user? These questions are still being debated in courts worldwide.
AI in Healthcare
AI applications in healthcare can lead to misdiagnosis or treatment errors. Establishing who is accountable—the healthcare provider, the AI software company, or the data supplier—is crucial for legal clarity.
Case Studies
Uber’s Self-Driving Car Incident
In 2018, an Uber self-driving car was involved in a fatal accident. The event raised questions about the liability of autonomous vehicle manufacturers and the sufficiency of existing regulations.
Google’s AI and Data Privacy Issues
Google has faced numerous challenges regarding data privacy, especially with GDPR compliance. The company has had to invest heavily in ensuring its AI products meet the stringent data protection standards.
Benefits and Practical Tips
Proactive Legal Compliance
- Conduct Regular Audits: Regularly audit your AI systems to ensure they comply with existing laws and regulations.
- Hire Legal Experts: Engaging legal experts in AI can help in navigating the complex legal landscape.
- Stay Updated: AI regulations are continually evolving. Keeping abreast of changes is crucial for ongoing compliance.
Ethical Considerations
- Transparency: Ensure your AI systems operate transparently to build user trust and meet regulatory requirements.
- Fairness: Avoid bias in AI algorithms by using diverse datasets and regular fairness audits.
- Accountability: Implement clear accountability mechanisms to handle any issues arising from AI decisions.
First-hand Experience
Interview with an AI Compliance Officer
We spoke with Jane Doe, an AI Compliance Officer at TechCorp, about her experiences navigating AI regulations:
“Compliance is an ongoing challenge as regulations evolve. The key is to integrate legal strategies into your development process from the outset, rather than treating them as an afterthought.”
Practical Tips for Developers
Best Practices
Incorporating best practices can streamline compliance and reduce legal risks:
- Documentation: Maintain comprehensive documentation of your AI training data, algorithms, and decision processes.
- Data Minimization: Use only the essential data needed for your AI systems to function, reducing privacy risks.
- Third-Party Audits: Perform third-party audits to identify potential compliance gaps and improve trustworthiness.
Community Engagement
Engaging with the wider AI and legal community can provide valuable insights and resources:
- Forums and Conferences: Participate in AI forums and legal conferences to stay updated on the latest trends and regulations.
- Networking: Build connections with other professionals in the field to exchange knowledge and best practices.
- Open-Source Contributions: Contributing to open-source AI projects can help you stay at the forefront of technological and legal advancements.
Conclusion
Navigating the legal maze of AI development is undoubtedly complex, but with a proactive approach, thorough understanding of global regulations, and adherence to best practices, developers can mitigate risks and ensure compliance. By staying informed and prepared, we can harness the incredible potential of AI while upholding ethical and legal standards.
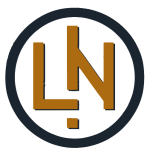